
Extensive experiments are implemented on the Vaihingen, Potsdam and Gaofen RSI datasets, where the comparison results demonstrate the superior performance of RSI-Net in terms of overall accuracy (91.83%, 93.31% and 93.67% on three datasets, respectively), F1 score (90.3%, 91.49% and 89.35% on three datasets, respectively) and kappa coefficient (89.46%, 90.46% and 90.37% on three datasets, respectively) when compared with six state-of-the-art RSI semantic segmentation methods.Ī deep neural network (DNN), evolved from a traditional artificial neural network, has been seamlessly adapted for the spatial data domain over the years. The first component explicitly models correlations between adjacent land covers and conduct flexible convolution on arbitrarily irregular image regions by using graph convolutional network, while densely connected atrous convolution network (DenseAtrousCNet) with multi-scale atrous convolution can expand the receptive fields and obtain image global information. In contrast to previous work that utilizes dilated convolutions or deep models, we propose a novel two-stream deep neural network for semantic segmentation of RSI (RSI-Net) to obtain improved performance through modeling and propagating spatial contextual structure effectively and a decoding scheme with image-level and graph-level combination.
0P F1 2019 IMAGE HOW TO
How to get the trade-off effectively is an open question, where current approaches of utilizing very deep models result in complex models with large memory consumption. We conclude that DCEC is a promising new method in landscape and land-system research.įor semantic segmentation of remote sensing images (RSI), trade-off between representation power and location accuracy is quite important. DCEC successfully distinguished 45 landscape classes in the continuous input data. We applied DCEC to image tiles extracted from satellite images as well as ecological, demographic and terrain layers. DCEC encodes lower-dimensional representations of input images in a hidden layer, which is simultaneously used to divide the images into well-distinguishable clusters. To unlock the great potential of continuous spatial data, such as remote sensing images, for generating landscape typologies, we adapted a novel unsupervised deep learning method (Deep Convolutional Embedded Clustering DCEC) to generate a landscape typology for Switzerland.
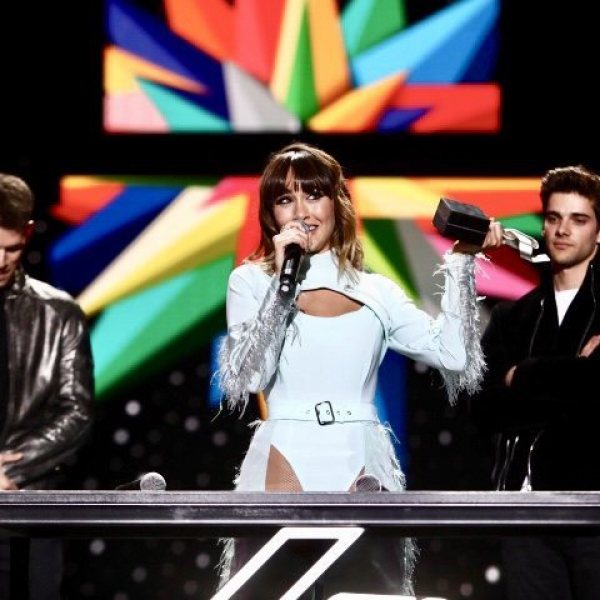
Although landscape patterns are key distinctive features of landscape classes, existing unsupervised clustering techniques for clustering landscapes rely on categorical input data to quantify such patterns and consider only a limited number of pattern metrics. The identification of landscape classes facilitates the implementation of planning strategies.
